Slag is the main by-product of iron and steelmaking, and significant efforts are being made to enhance its reuse and recycling. The EU-funded iSlag project focused on optimizing slag valorization through digital solutions. As a key partner, Tenova developed and tested the Tenova LIBS system for rapid slag analysis. By providing real-time data on slag composition, the project improved recycling control, reduced environmental impact, and made slag recovery more efficient and cost-effective.
Slag represents around 90% of all steelmaking by-products by mass. It is usually a mixture of metal oxides, including calcium and magnesium, as well as silicon dioxide. It can also contain metal sulfides and elemental metals. Its disposal at landfills wastes valuable resources and if slag is properly valorized, it can play a key role in the circular economy of the steel industry.
The EU Green Deal has set ambitious targets to achieve climate neutrality by 2050, and the decarbonization of the steel industry, which is responsible for around 5% of CO2 emissions in the EU and 7% globally, is pivotal to meeting these goals. One of the main building blocks of the Green Deal is the circular economy action plan, which seeks to reduce pressure on natural resources while at the same time creating sustainable and financial growth.
This is where the valorization of slag comes in: if the steel industry treats it as a valuable raw material, increasing its life cycle, it can reduce waste, lower carbon emissions and facilitate the decarbonization of this hard-to-abate sector.
It is in this context that iSlag, an EU project funded by the Research Fund for Coal and Steel, was conceived, and Tenova joined as a technology partner. Taking place over four years (2020-2024), the project’s aim was to develop novel digital solutions to optimize the reuse and recycling of slag produced by the electric arc furnace (EAF) and ladle furnace (LF) processes.
The general objectives of iSlag included:
- The creation and implementation of advanced devices capable of real-time, accurate characterization of both liquid and solid slag in industrial settings.
- The exploration of optimal recycling and post-treatment pathways for different types of slag, facilitating sustainable practices within the steel industry.
- The development of comprehensive models to predict the composition and volume of slag based on data from characterization devices, aiding in the optimization of metallurgical processes.
- Establishment of Decision Support System (DSS) to assist technical personnel in making informed decisions about slag treatment and reuse, enhancing the efficiency and sustainability of steel production.
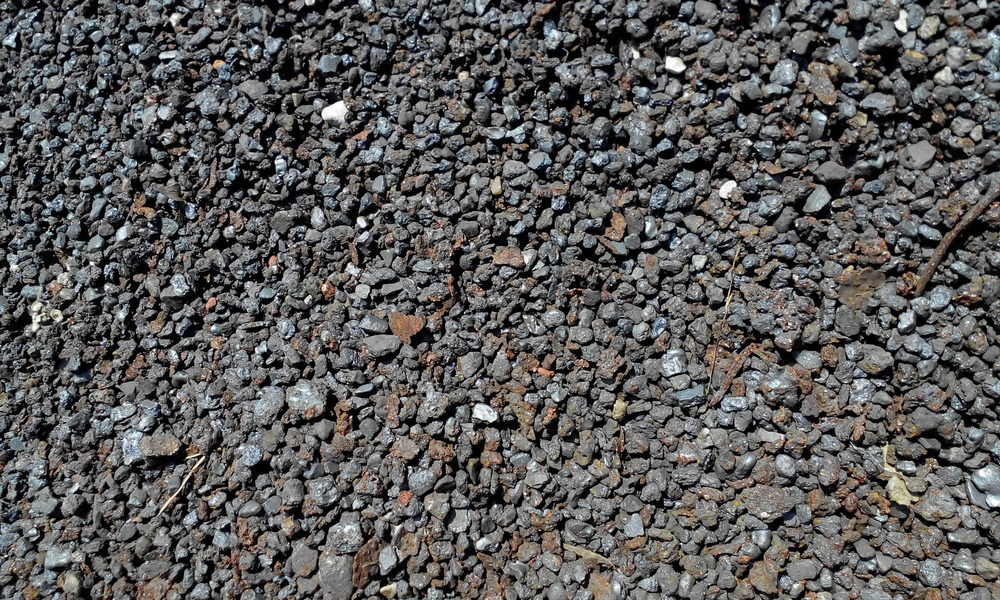
Tenova played a crucial role in achieving these objectives by leveraging its expertise in metallurgical processes and industrial automation. Our responsibilities included designing and implementing the diagnostic systems for real-time slag analysis. Crucially, we developed and field tested the Laser Induced Breakdown Spectroscopy (LIBS) system.
LIBS is a powerful analytical technique used to determine the composition of materials by focusing a high-energy laser pulse onto the surface of a sample.
The system doesn’t require any sample preparation or prior calibration procedure, allowing for rapid characterization of steel and slag samples with the same device.
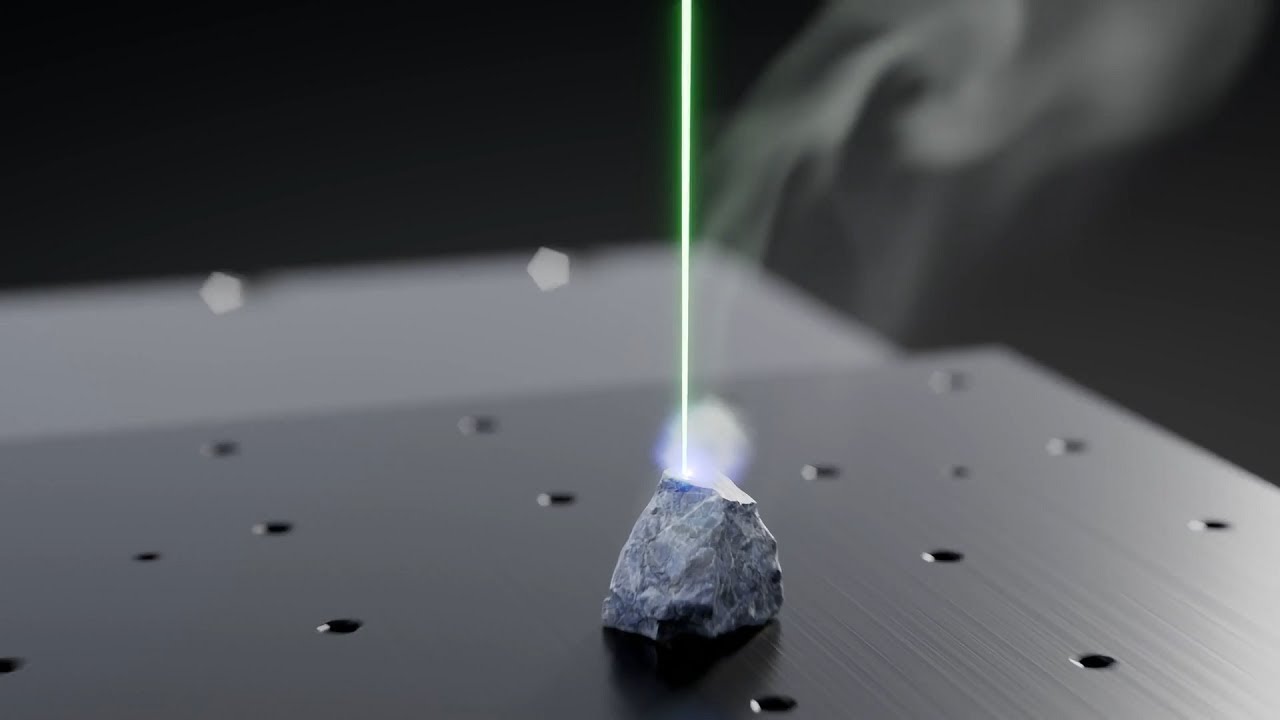
To enhance the accuracy of the LIBS system, Tenova also developed a Machine Learning (ML) workflow, which processes the data collected by LIBS. By using advanced algorithms like Support Vector Regression (SVR) and Extreme Gradient Boosting Regression (XGBR), the ML workflow delivers precise and reliable predictions. The models were trained on extensive datasets collected from field tests (one model per element) and were in fact consistently able to provide reliable predictions, even in the challenging environments of steel production facilities.
The LIBS system was successfully tested at TenarisDalmine’s EAF meltshops and Sidenor Aceros Especiales Basauri plants. The positive results underscored the system's capability to provide rapid, accurate slag composition analysis, supporting efficient and sustainable steel production practices. The detailed composition analysis provided by the LIBS system facilitates the identification of optimal recycling and reuse pathways, maximizing the economic value derived from slag.
Laser Induced Breakdown Spectroscopy (LIBS) works by focusing a high-energy laser pulse onto the surface of a sample, creating a plasma plume. This plasma emits light at characteristic wavelengths corresponding to the elements present in the sample. The emitted light is collected and further analyzed using a spectrometer that separates the light into its component wavelengths. By measuring the intensity of these wavelengths, the LIBS system can accurately quantify the concentration of various elements in the sample. This method is highly advantageous because it requires minimal sample preparation, can analyze multiple elements simultaneously, and provides results in real-time, making it ideal for industrial applications.
Machine Learning workflow processes data collected by LIBS and uses ML modeling, including Support Vector Regression (SVR) and Extreme Gradient Boosting Regression (XGBR), to deliver precise and reliable predictions. The preprocessing stage of the workflow consists of a set of sequential transformations that take raw spectrum data and transform it to the model-ready set of inputs. This stage includes steps such as spectrum alignment, outlier filtering, averaging, normalization, and background. The preprocessed data is then used to either train models or generate predictions. SVR is a powerful and versatile ML technique capable of handling linear and non-linear regression problems. It is particularly suitable for small- and medium-sized datasets. XGBR is a robust and efficient boosting algorithm that has the ability to achieve high performance in many learning tasks with both small and large datasets.
The iSlag project exemplifies how digital innovations can support the circular economy in heavy industries. By improving the efficiency of slag recycling, Tenova’s LIBS system allows to decrease landfill waste, and helps lower carbon emissions - advancing the steel industry towards a greener future.
Want to know more?
Get in touch with our experts on the subject